So get ready to make your database faster and more cost-effective!
Key Takeaways
- Understand Snowflake’s pricing model, which includes storage and compute costs, to effectively optimize Snowflake Virtual Warehouses.
- Implement warehouse size optimization and workload optimization by grouping similar workloads, leveraging data SLAs, right – sizing utilization, and setting up resource and volume monitors in Snowflake.
- Use query tags to identify expensive queries and make necessary optimizations for improved speed in Snowflake.
- Utilize table optimization techniques such as search optimization service or table clustering to maximize data storage efficiency and enhance response.
Understanding Snowflake Cost Optimization in 2024
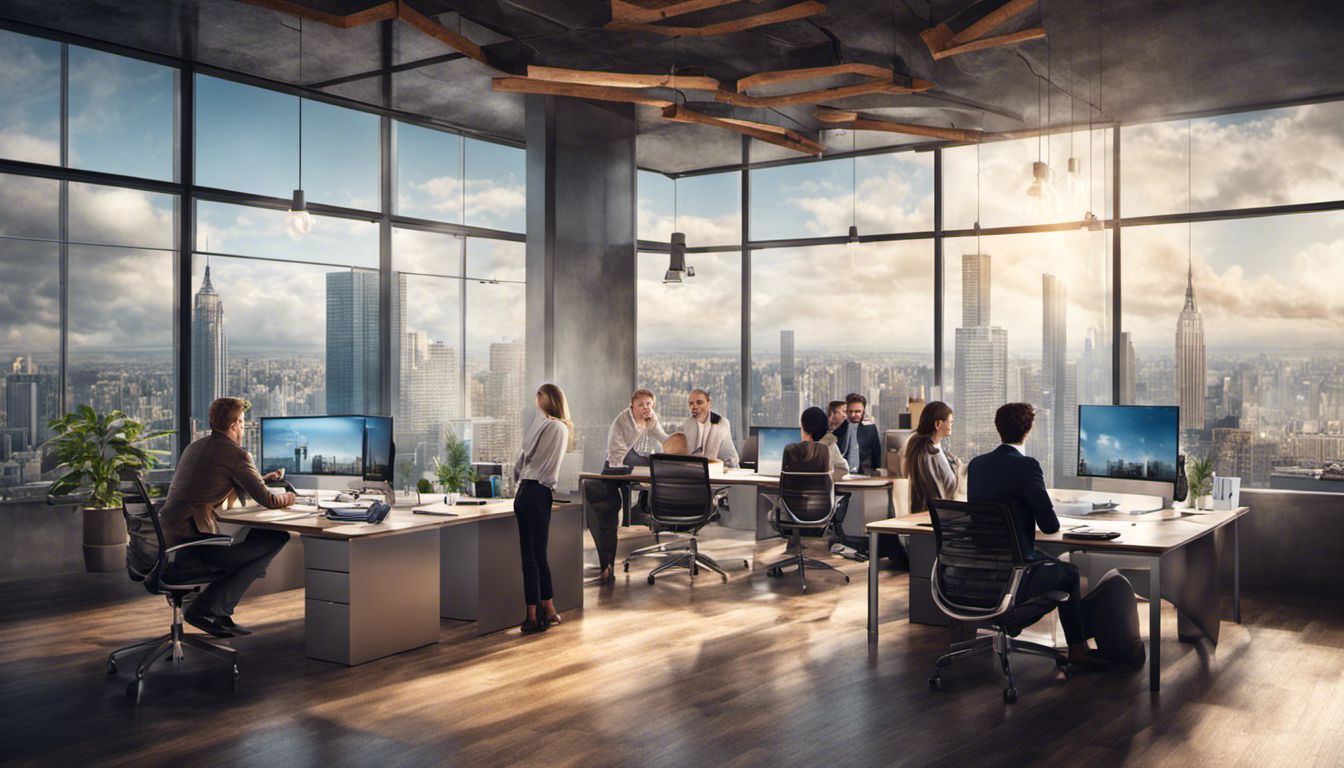
To effectively use Snowflake in 2024, it is important to understand its pricing model and implement steps such as warehouse size optimization, query optimization, and table optimization.
Snowflake pricing model
Snowflake adopts a consumption-based pricing model, allowing you to pay only for the resources you use. It divides costs into two components: storage and compute. Storage cost depends on the amount of data stored in Snowflake, billed on a per-terabyte basis.
For computing, it charges based on the time your virtual warehouses run, designated as credits. This flexible approach encourages efficient resource usage while keeping expenses manageable.
You can adjust your warehouse size or suspend it when not in use to control costs effectively. Plus, Snowflake also offers additional options for committed usage which could lead to savings over time.
Steps for cost optimization: warehouse size, query optimization, table optimization
Analyzing Snowflake for reduced costs and improved performance is a crucial task. The process involves three key steps to streamline your efforts:
- Warehouse Size Optimization: Select the most efficient warehouse size that matches your usage pattern to minimize costs. With the use of optimization techniques, you can leverage data SLAs to set appropriate resource and volume monitors. It helps in proper utilization and efficient handling of similar workloads.
- Query Optimization: You need to consistently analyze and improve response time. Achieve this by employing tags for each query, which aids in identifying and managing expensive or heavy queries effectively. It is imperative for data engineers SQL calls to receive an immediate response.
- Table Optimization: Enhance the speed and efficiency of searches with table optimization. Decide between using an optimization service or table clustering based on your unique needs. Regularly review and eliminate unused tables to maintain a lean database system.
Warehouse Size Optimization
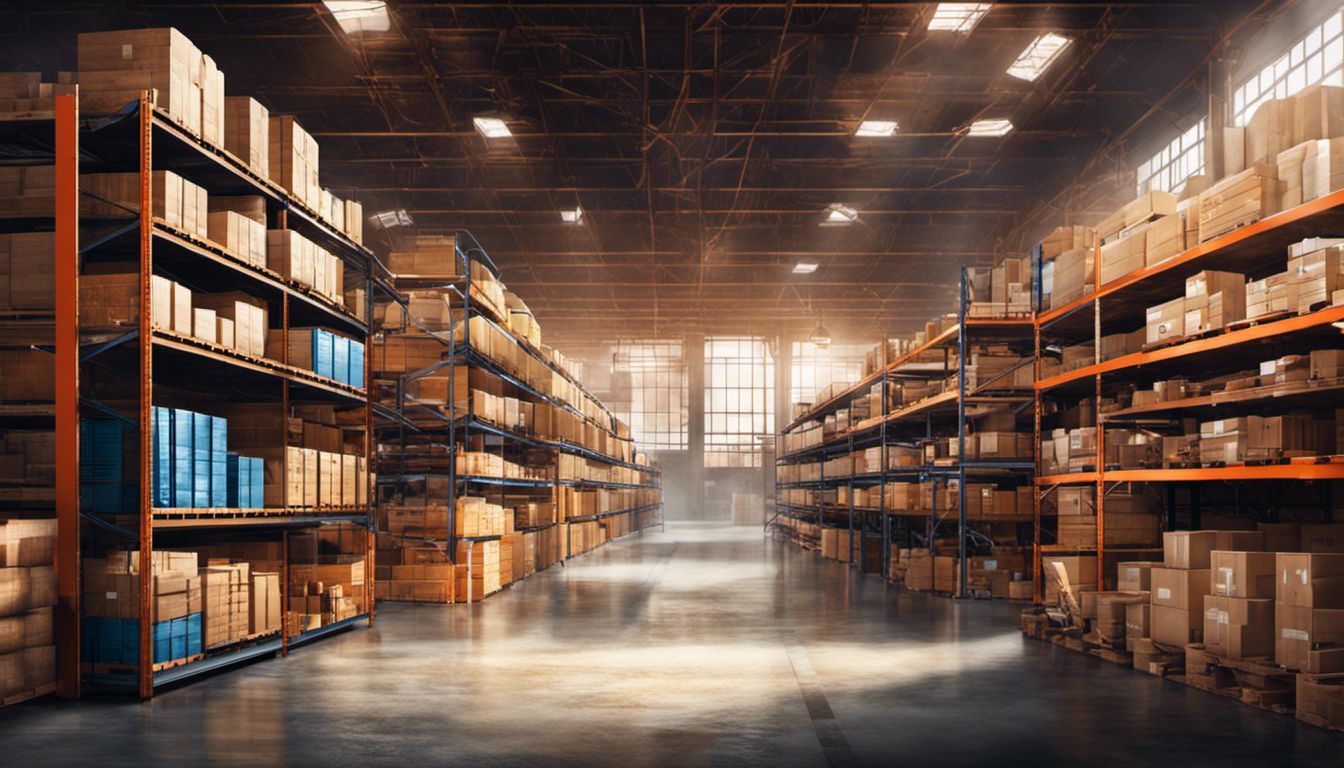
To improve your Snowflake warehouse size, start by grouping similar workloads and leveraging data SLAs to ensure resources are allocated efficiently. Next, right-size utilization by monitoring resource and volume usage and making adjustments as needed.
By following these steps, you can improve warehouse size for Snowflake usage.
Grouping similar workloads
Grouping similar workloads effectively maximizes your optimization efforts. By doing this, you ensure that the same kind of tasks are running together, thus increasing efficiency and reducing runtime.
This approach lowers costs as it reduces the amount of compute resources needed to process disparate workloads at varying intervals. It’s like organizing a marathon; runners with similar skill levels start together making tracking progress simpler and more efficient than if all different level participants were mixed up.
Just make sure to tune your warehouse sizing according to these grouped workloads for optimal performance tuning.
Leveraging data SLAs
Leveraging data service level agreements (SLAs) can be a powerful way to maximize your Snowflake data. SLAs define the expected level of service for different types of data, such as high-priority or low-priority data.
By prioritizing queries based on these SLAs, you can ensure that critical queries get processed quickly while less important ones are given lower priority. This helps to improve resource allocation and improve overall system response in Snowflake.
By effectively leveraging data SLAs, you can achieve better efficiency and cost savings in your Snowflake environment without compromising on performance.
Right-sizing utilization
When evaluating the cost and performance of Snowflake, it’s important to right-size utilization. This means efficiently allocating resources to match the workload requirements. By closely monitoring your warehouse usage, you can identify any underutilization or overutilization issues.
For example, if your workload is consistently exceeding the allocated capacity, you may need to scale up the warehouse size for better performance. On the other hand, if your usage is consistently low, downsizing the warehouse can help reduce costs.
Regularly analyzing and adjusting resource allocation based on actual needs will ensure optimal utilization of Snowflake and help maximize both performance and cost efficiency.
Setting resource and volume monitors
It is important to set up resource and volume monitors. By doing so, you can closely monitor your database usage and identify any potential bottlenecks or areas of improvement. A dashboard that displays this information is recommended.
Resource monitors allow you to allocate resources based on workload priority, ensuring that critical queries are given the necessary resources for efficient execution. Volume monitors help track data storage usage, allowing you to manage storage costs effectively by identifying unused or redundant data that can be removed.
With these monitors in place, you can proactively manage your Snowflake environment and make informed decisions for better performance and cost optimization.
Query Optimization
When analyzing the Snowflake query optimization, you can use query tags to identify expensive queries and improve their execution. Want to learn more about how to effectively improve your queries? Keep reading!
Using query tags
To improve query performance, you can use query tags. Query tags allow you to label and categorize your queries based on different criteria such as business unit, project, or priority.
By assigning query tags to your queries, you can easily track and analyze their performance and resource consumption. This helps you identify any expensive or heavy queries that may be impacting the overall system performance.
With this information, you can then make necessary optimizations to improve query performance and reduce costs.
Identifying expensive and heavy queries
It is crucial to identify expensive and heavy queries. By doing so, you can pinpoint areas where improvements are needed. One way to accomplish this is by utilizing query tags, which provide a mechanism for categorizing and tracking different types of queries.
This allows you to easily identify the most resource-intensive queries and take steps to improve them. Additionally, reviewing query history and analyzing execution times can help isolate specific queries that may be impacting overall performance or incurring high costs.
By identifying these expensive and heavy queries, you can make targeted optimizations that will enhance both the speed and cost-effectiveness of your Snowflake environment without compromising functionality or data integrity.
Table Optimization
Table optimization plays a crucial role in determining performance and cost. By utilizing search optimization services and identifying unused tables, you can maximize the efficiency of your data storage and improve overall performance.
Find out more about these techniques in our blog post!
Search optimization service vs table clustering
When it comes to Snowflake, two critical methods can be used in system optimization; Search Optimization Service and Table Clustering. The two differ in their approach and advantages they provide.
Search Optimization Service | Table Clustering |
Search optimization service uses algorithms to quickly locate and return requested data | Table clustering organizes data based on defined criteria to increase data retrieval efficiency |
It is ideal for databases with heavy read operations since it enhances search speed | It is suitable for databases with heavy write operations, as it improves data storage for quicker updates |
It does not require a specific arrangement of data, making it flexible for diverse data types | It requires a specific column to cluster the table around, hence may not be suitable for all data types |
It offers reduced search time and lesser system resources, thereby reducing costs | It improves write operations efficiency, minimizing system lag and potential downtime costs |
Understanding these two techniques and their benefits can help you make informed decisions on which strategy best suits your database needs for a cost-effective and efficient system.
Unused tables
Unused tables in Snowflake can have a significant impact on both performance and cost. These are tables that are no longer being used or accessed by any queries in your database. By identifying and removing these unused tables, you can improve your Snowflake environment for better response and reduce unnecessary costs.
It’s important to regularly review your database to identify any tables that may be obsolete or outdated. Removing these unused tables will not only free up storage space but also improve the overall efficiency of your queries and data processing.
Conclusion
In conclusion, analyzing Snowflake for performance and cost is crucial for technology professionals with database responsibilities. By carefully managing warehouse size, implementing query optimization techniques, and improving table usage, you can improve system performance and reduce costs.
Take advantage of Snowflake’s features like query tags and search optimization service to further optimize your Snowflake environment. With these strategies in place, you can maximize the efficiency of your Snowflake setup while minimizing expenses.
FAQs
Q: What is Snowflake Optimization for Performance and Cost?
A: Snowflake Optimization for Performance and Cost refers to the techniques and strategies used to maximize the performance and minimize the cost of running queries on Snowflake, a popular cloud-based data warehouse platform.
Q: How can I accelerate my Snowflake queries?
A: There are several ways to speed-up Snowflake queries, such as improving the query structure, using appropriate data types, creating materialized views, partitioning tables, pruning unnecessary data, and leveraging query optimization features provided by Snowflake.
Q: What is a materialized view in Snowflake?
A: A materialized view in Snowflake is a precomputed and stored result of a query that can be used for fast retrieval of data. It helps to improve query performance by reducing the need for executing the underlying query each time data is requested.
Q: How can I create a materialized view in Snowflake?
A: To create a materialized view in Snowflake, you need to define the view using a SELECT statement and specify the desired refresh schedule and storage options. Snowflake will automatically maintain and update the materialized view based on the defined schedule.
Q: What is a virtual warehouse in Snowflake?
A: A virtual warehouse in Snowflake is a compute resource that is dedicated to executing queries. It provides the necessary processing power and memory to handle query workloads efficiently. Virtual warehouses can be scaled up or down based on the workload requirements.
Q: How can I improve the performance of my virtual warehouses in Snowflake?
A: You can improve the performance of virtual warehouses in Snowflake by selecting an appropriate size based on your workload requirements, monitoring resource usage using the Snowflake Resource Monitor, and tuning queries to take advantage of parallel processing capabilities offered by Snowflake.
Q: What is Snowflake’s auto-suspend feature?
A: Snowflake’s auto-suspend feature allows you to automatically suspend virtual warehouses when they are idle for a specified period of time. This helps to reduce costs by freeing up compute resources when they are not in use.
Q: How can I ensure data quality in Snowflake?
A: To ensure data quality in Snowflake, you can implement data validation checks and constraints, perform regular data cleaning and profiling, establish data governance processes, and leverage Snowflake’s built-in features for data validation and quality monitoring.
Q: What are some best practices for reducing Snowflake costs?
A: Some best practices for reducing Snowflake costs include improving query performance to minimize compute resource usage, using appropriate virtual warehouse sizes based on workload requirements, enabling auto-suspend for idle virtual warehouses, managing and monitoring Snowflake credits, and leveraging caching and data pruning techniques.
Q: How can I monitor the performance of my Snowflake queries?
A: You can monitor the performance of your Snowflake queries by analyzing query profiles, monitoring the resource usage of virtual warehouses, using Snowflake’s built-in observability features, and setting up alerts and notifications for critical performance metrics.